AI Is About To Take Cybersecurity By Storm: Here's What You Can Expect
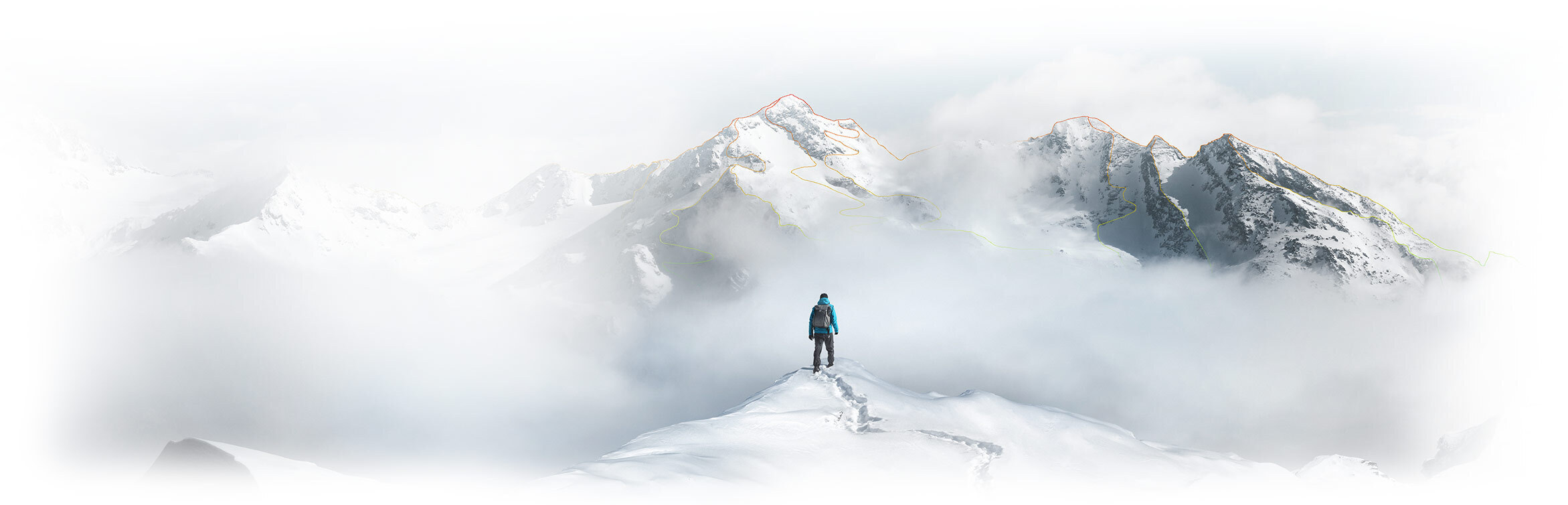
Generative AI will elevate the practice of successful preventive cybersecurity, but how will it manifest itself across cybersecurity products? Here are a few game-changers to look for.
Recent breakthroughs in generative artificial intelligence (AI) tools like ChatGPT have sparked a firestorm of debate about how to harness the power of the technology for good while minimizing its potential for harm. In cybersecurity, the promise of generative AI is leading organizations of all sizes to evaluate, experiment and engage with AI in new and exciting ways — and generating an awful lot of noise in the process. Making sense of it all can be daunting, but it all boils down to two key factors: a revolutionary new user experience and a spotlight on data quality.
A new cyber UX
In my view, the reason the technology world is getting excited about AI is because, for the last 60 years, we've had the same experience on computers. Of course, user experience has improved in that time, but we’re still operating with the same basic structure: command line, windows, files and widgets. Machine learning and AI have already revolutionized the way systems operate behind the screen. Generative AI is going to change the way humans interact with software, computing devices and the cloud. Asking questions just as we would address another human being will become our new user interface and search tool, making using a computer as easy as talking to a person — albeit one who has all the patience in the world.
Driving cyber efficiency and productivity
When it comes to sectors like cybersecurity, which is already saddled with a skills deficit, generative AI can be a force multiplier, opening up new career opportunities for professionals who would not have previously been able to serve without assistance. It is also about raising the game — everybody’s game — in cybersecurity. Because, right now, cyber defenders are not always winning the war. According to a commissioned study of 825 security and IT professionals conducted by Forrester Consulting on behalf of Tenable, nearly six in 10 respondents (58%) say the security team is too busy fighting critical incidents to take a preventive approach to reduce their organization’s exposure. The vast majority (73%) believe their organization would be more successful at defending against cyberattacks if they could devote more resources to preventive cybersecurity.
AI strategy rhymes with data strategy
AI has the potential to tilt the odds in favor of defenders, but it is only as effective as the data it’s built on. AI and data are the yin and the yang. If you have unique data then you’re going to have unique intelligence guiding your decisions. It’s truly “garbage in, garbage out” — or “gold in, gold out” — depending on your sources. It all starts with gathering your cyber data. According to the Forrester study, most organizations have to pull data from at least nine different sources, including cloud findings; threat intelligence feeds; incident-readiness assessment findings; vulnerability disclosures; penetration test findings; and external attack surface findings. To be truly effective in the practice of preventive cybersecurity, AI requires breaking down silos to enable defenders to take the data from their mix of multiple cybersecurity point solutions and use it to create something wholly new. And they have to rely on multiple systems/methods to pull all that data together: aggregation tools, internal data lake, and the old reliable multi-tabbed spreadsheet. For all these reasons, data integration is the essence of the Tenable One Exposure Management Platform. The approach is as simple as it is powerful: Let us bring all preventive security data into one single data lake so we can prioritize, contextualize and apply generative AI.
AI game-changers to look for in cybersecurity
Now, when it comes to using AI in cybersecurity, what can we expect? You can definitely expect a “gold rush” from your favorite security vendors. Everyone is succumbing to the siren's song of large language models (LLM). Watch for the hype: machine learning is a subfield of AI, and traditional AI is not generative AI. So, what will emerge from the early adoption of generative AI across security products and vendors? Here are three simple design patterns to look for in any AI-powered cybersecurity solution:
- Does it offer natural-language search? Major security categories — such as preventive exposure management, security information and event management (SIEM), extended detection and response (XDR), and user and entity behavior analytics (UEBA) — are already data-centric. With so many data points to analyze, effective search is critical. The potential for AI to deliver natural language-based search functionality is a game changer for cybersecurity professionals. For example, imagine it is December 13, 2021, and you’re a vulnerability management analyst. Log4Shell was recently disclosed, affecting instances of Apache Log4j everywhere. According to Tenable telemetry, in December 2021 one in 10 assets was vulnerable to Log4Shell, including a wide range of servers, web applications, containers and IoT devices. Such zero-day disclosure is a big deal. You'd need to drop everything and get to work — you'd be on the hunt to find Log4j across your disparate environments. If you had an exposure management solution, it would have already collected all the data across your IT, OT and public cloud environments. Somewhere in that data lake would lie the answer you are looking for: “Where is log4j in my environment?” Finding all these instances would require formulating a complex query in a cryptic query language and scrambling to digest the details of a vendor’s data model. If you had the additional power of LLM, you'd no longer need to be a master of complexity. You would simply type ”Show me where I have Log4j across my IT, OT and Amazon Web Services (AWS) cloud?” and voila! Science fiction? Not at all. This is the art of the new AI possible.
- Does it explain? We all know this: Cybersecurity is hard. It takes years to fully train security professionals across the myriad of tools and disciplines of cybersecurity. Generative AI can explain, in plain and simple words, the particulars of a given vulnerability. It can explain its criticality and how it would be exploited by an attacker. It can provide clear guidance on each step along the way to fixing it. It can explain a new advanced persistent threat (APT) or an indicator of compromise (IoC) alert popping up in the security operations center (SOC) at 1:00 a.m., when an exhausted security analyst has little time and context to decide whether the alert is a false positive or needs to be escalated for hunting. Any experienced security analyst would realize great value from AI. Instead of being overwhelmed by security issues, they’d be able to quickly churn through all the vulnerabilities and exposures and automate much of their response. But that’s not all. In addition to easing the challenges faced by seasoned security analysts, the ability of generative AI to explain everything cyber opens up opportunities for those who have not been educated in cybersecurity to now play a role. They’d have a co-pilot to guide them, explain what they're looking at, teach them and ultimately make them more productive. That’s a boon for everyone in cybersecurity.
- Does it guide action? A well-trained AI tool can act as a de facto assistant — a “sherpa” if you will. Soon, I would expect every cyber product to be accompanied by a chatbot. This cyber assistant, or co-pilot or sherpa, would steer you to identify the right actions to take across the varied terrain of specialized cyber solutions by answering any question in any language. Prompt-based interfaces, powered by vendor-specific data and intelligence built on LLM, using embeddings or fine-tuning, will indeed be the norm, making it quicker and easier for users to prioritize their remediation decisions. Indeed, the likes of Google Virtex AI, OpenAI GPT-4, LangChain and many others have made such capabilities accessible to any developer. The user experience of cybersecurity is about to change forever.
Conclusion
AI has the potential to change how cybersecurity professionals search for patterns, how they explain what they’re finding in the simplest language possible, and how they decide what actions to take to reduce cyber risk. The next phase of AI development will be even more compelling. Specialized cyber models will be capable of finding patterns and anomalies in your cyber data on their own and at superhuman speeds. They will also be capable of quickly identifying and automating critical actions that need to be taken. These capabilities will make preventive cybersecurity possible at scale. With the support of AI, preventive cybersecurity practices will become increasingly effective, perhaps someday enabling defenders to stay one step ahead of attackers.
Learn more
- Download the Tenable Research report, How Generative AI Is Changing Security Research
- Exposure Management
- Exposure Management